海归学者发起的公益学术平台
分享信息,整合资源
交流学术,偶尔风月
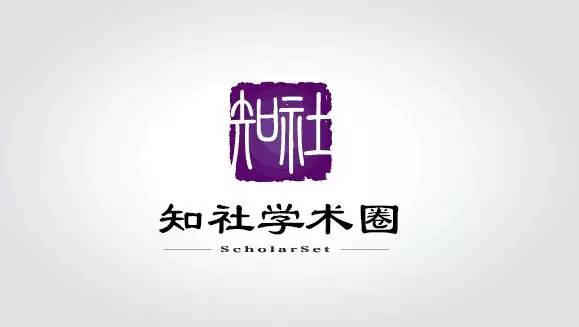
宏观对称性是现代凝聚态物理和材料科学的核心概念之一。与无序系统相比,基于对称性的描述符让人们对具有平移对称性材料的结构和功能有了更深入的了解。至21世纪以来,高分辨率电子和扫描探针显微镜成像的出现,为获取体材料、二维材料和表面的原子分辨率图像打开了闸门。这些图像中的海量数据包含了与材料结构、结构变形和物理功能相关的信息。掌握这些信息,需要开发用于提取相关信息的数学框架。然而,迄今针对原子分辨图像的分析,通常都是基于对宏观物理学概念的运用,如平移、点群对称性、对称性降低等。在这一点上,显微测量的本质是根本不同的。
来自美国橡树岭国家实验室纳米材料科学中心的Sergei V. Kalinin(本刊副主编)研究团队,提出了一种基于贝叶斯的深度学习方法来分析空间解析数据。该方法利用了三个基本概念的协同作用,即对应于稳定原子构型的原子级描述符的简约,理想化描述符中存在的扭曲,和可能存在的离散或连续性旋转对称。这三个概论在一个工作流中实现,结合了特征选择(原子查找),一个旋转不变变分自动编码器,以及一个条件自动编码器。该方法适用于2D图像数据,但也可以推广到更复杂的多维数据集。作者提出的工作流程,不仅能对原子分辨 STEM 成像数据进行自下而上的对称性和结构分析,还为研究材料系统中的对称破坏畸变开辟了道路。该文近期发表于npj Computational Materials 7, 181 (2021),英文标题与摘要如下,点击左下角“阅读原文”可以自由获取论文PDF。
Deep Bayesian local crystallography
Sergei V. Kalinin, Mark P. Oxley, Mani Valleti, Junjie Zhang, Raphael P. Hermann, Hong Zheng, Wenrui Zhang, Gyula Eres, Rama K. Vasudevan & Maxim Ziatdinov
The advent of high-resolution electron and scanning probe microscopy imaging has opened the floodgates for acquiring atomically resolved images of bulk materials, 2D materials, and surfaces. This plethora of data contains an immense volume of information on materials structures, structural distortions, and physical functionalities. Harnessing this knowledge regarding local physical phenomena necessitates the development of the mathematical frameworks for extraction of relevant information. However, the analysis of atomically resolved images is often based on the adaptation of concepts from macroscopic physics, notably translational and point group symmetries and symmetry lowering phenomena. Here, we explore the bottom-up definition of structural units and symmetry in atomically resolved data using a Bayesian framework. We demonstrate the need for a Bayesian definition of symmetry using a simple toy model and demonstrate how this definition can be extended to the experimental data using deep learning networks in a Bayesian setting, namely rotationally invariant variational autoencoders.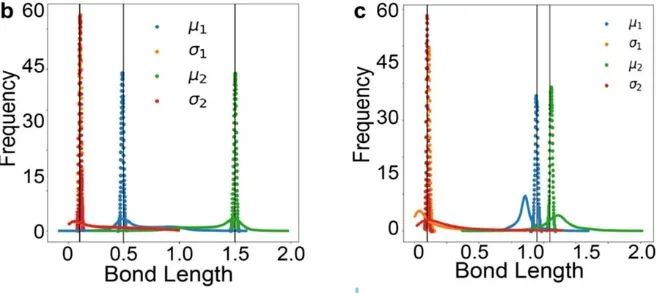