海归学者发起的公益学术平台
分享信息,整合资源
交流学术,偶尔风月
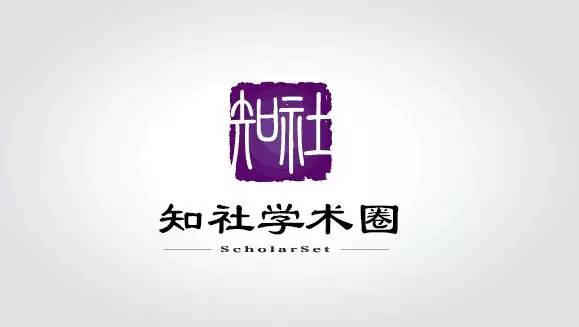
沸石具有多样化的结构和性质,在工业领域有多方面应用,如催化、吸附以及离子交换等。为加快沸石的搜索和研发,计算机模拟必不可少,但针对结构和性能的筛选仍存在一定挑战。近年来,机器学习在材料科学和化学领域中的得到广泛应用,这使人们有望理解沸石结构、稳定性和性质之间的复杂相关性。然而,在原子水平上生成准确的结构和能量数据,是找到可靠相关性的先决条件。从头计算虽然能提供足够准确的结构与能量,但是真实沸石结构的建模与模拟需要昂贵的计算资源。因此,至关重要的一点是,需要进行具有从头计算精度的大规模模拟。迄今,已有的机器学习势(MLP)还不能综合考虑到沸石的结构多样性以反应相变,这都为沸石的精确大规模模拟带来了困难。
来自查理大学物理与高分子化学系的Lukáš Grajciar教授团队,基于密度泛函理论(DFT)数据,使用SchNet架构进行了神经网络势(NNP)训练。通过NNPs的主动学习,他们扩展并完善了沸石数据库,包括低能平衡态与高能过渡态结构。他们利用训练后的NNPs对沸石数据库进行了再优化,在沸石合成的热力学可及范围内额外发现了超过20000种沸石结构。此外,经过严格的精度测试,NNPs不仅与从头计算、实验结果具有良好的一致性,而且精度比其他势能面近似方法高出一个数量级。该项工作为硅质沸石的设计与合成提供了一种准确的计算方法。
该文近期发表于npj Computational Materials 8:174(2022),英文标题与摘要如下,点击左下角“阅读原文”可以自由获取论文PDF。
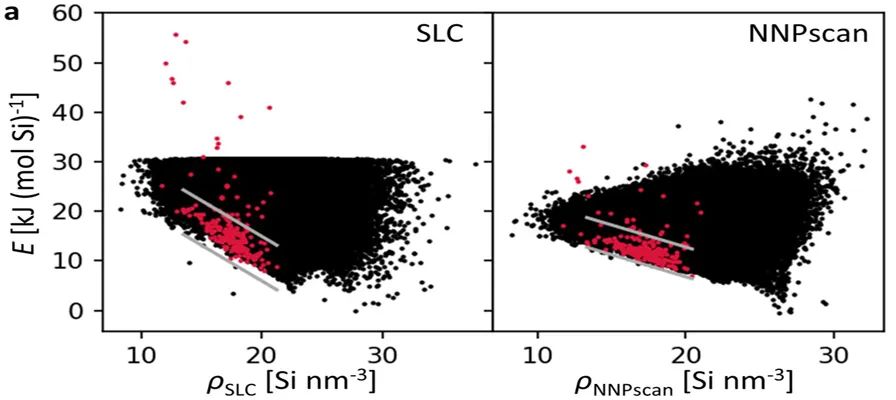
Accurate large-scale simulations of siliceous zeolites by neural network potentials
Andreas Erlebach, Petr Nachtigall & Lukáš Grajciar
The computational discovery and design of zeolites is a crucial part of the chemical industry. Finding highly accurate while computational feasible protocol for identification of hypothetical siliceous frameworks that could be targeted experimentally is a great challenge. To tackle this challenge, we trained neural network potentials (NNP) with the SchNet architecture on a structurally diverse database of density functional theory (DFT) data. This database was iteratively extended by active learning to cover not only low-energy equilibrium configurations but also high-energy transition states. We demonstrate that the resulting reactive NNPs retain DFT accuracy for thermodynamic stabilities, vibrational properties, as well as reactive and non-reactive phase transformations. As a showcase, we screened an existing zeolite database and revealed >20k additional hypothetical frameworks in the thermodynamically accessible range of zeolite synthesis. Hence, our NNPs are expected to be essential for future high-throughput studies on the structure and reactivity of siliceous zeolites.
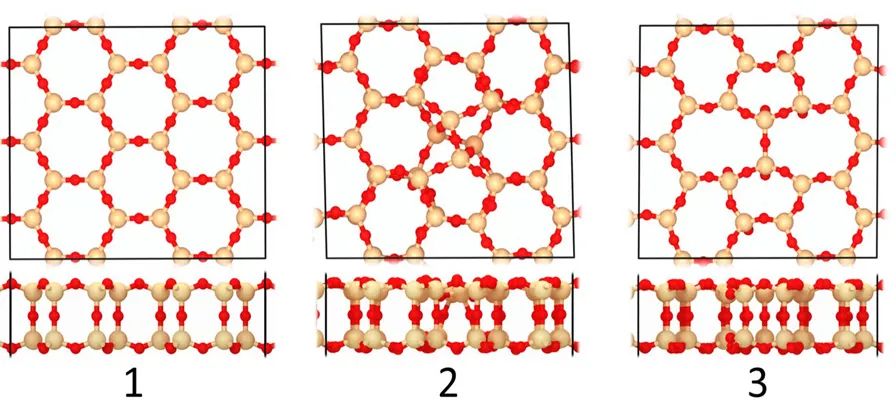